Over the past several months we’ve been working on improving matchmaking. In this post we’d like to share with you where matchmaking currently stands and give you a sneak peek on an upcoming matchmaking feature.
Ranked Matchmaking is Coming
The next major update will add a ranked matchmaking feature to the game. This mode is aimed at experienced players who want to play in a more competitive environment and know their matchmaking rating (MMR). Dota 2 matchmaking has always calculated MMR and used it to form matches; in ranked matchmaking we make that MMR visible.
Here’s what you need to know about ranked matchmaking:
- Ranked matchmaking is unlocked after approximately 150 games.
- All players in the party must have unlocked the mode.
- Currently, only All Pick, Captains Mode, and Captains Draft are available.
- You may not participate in ranked matchmaking while in the low priority pool.
- Coaches are not allowed in ranked matchmaking.
- Matches played in normal matchmaking do not impact your ranked matchmaking MMR, and vice versa.
- Your ranked MMR is visible only to you and your friends. The MMR used for normal matchmaking is not visible.
- When you first start using ranked matchmaking, you will enter a calibration phase of 10 games. During this time, your ranked MMR will not be visible.
Your Matchmaking Rating (MMR)
Dota 2 uses standard techniques to quantify and track player skill. We assign each player an MMR, which is a summary metric that quantifies your skill at Dota 2. After each match, we update your MMR based on what happened in that match. In general, when you win, your MMR will go up, and when you lose, your MMR will go down. Win/loss is the primary criteria used to update MMR, but individual performance also plays a role, especially when our uncertainty about your MMR is high. It is possible for an individual MMR to increase after a loss or decrease after a win, but in general the winning team’s average MMR will increase and the losing team’s MMR will decrease.
We also track our uncertainty about your MMR. New accounts and those playing in Ranked Matchmaking for the first time have high uncertainty. Higher uncertainty allows larger adjustments after each match, and lower uncertainty leads to smaller adjustments. Together, the MMR and uncertainty can be interpreted as a probability distribution of performance in your next game; the MMR itself serves as the mean of this distribution and the uncertainty is its standard deviation. If the match outcomes (both the win/loss and individual performance) repeatedly match our expectations, the uncertainty tends to decrease until it reaches a floor. A surprising match outcome will tend to cause an increase in uncertainty.
We actually track a total of four MMRs for each player:
- Normal matchmaking, queuing solo
- Normal matchmaking, queuing with a party
- Ranked matchmaking, queuing solo
- Ranked matchmaking, queuing with a party
Each of the two ranked MMRs has its own calibration period. Under certain circumstances, we may need to reactivate calibration, if we think the MMR is inaccurate.
To give you a feel for the range of MMR, below are some MMRs corresponding to various percentiles.
5% 1100
10% 1500
50% 2250
25% 2000
75% 2731
99% 4100
90% 3200
95% 3900
Note that this distribution is from normal matchmaking. We don’t know yet what the distribution will be in ranked matchmaking, but we expect it to be different. The players who participate in ranked matchmaking will be more skilled, more experienced players. We anticipate that any given player will have different expectations and play the game differently in ranked matchmaking compared to normal matchmaking.
What Makes a Good Match?
The ultimate goal of automated matchmaking in Dota 2 is for players to enjoy the game. The matchmaker seeks matches with the following properties (listed in no particular order):
- The teams are balanced. (Each team has a 50% chance to win.)
- The discrepancy in skill between the most and least skilled player in the match is minimized. This is related to team balance, but not the same thing.
- The discrepancy between experience (measured by the number of games played) between the least experienced player and the most experienced player is minimized. More on this below.
- The highest skill Radiant player should be close to the same skill as the highest skill Dire player.
- Each team contains about the same number of parties. For example, the matchmaker tries to avoid matching a party of 5 against against 5 individual players.
- Players’ language preferences contains a common language. Lack of a common language among teammates’ language preferences is strongly avoided. Lack of a common language across the whole match is also avoided, but less strongly.
- Wait times shouldn’t be too long.
The matchmaker seldom achieves all of those goals perfectly. For any potential match, the matchmaker assigns a quality score for each of the criteria above and then takes a weighted average. When the overall quality score exceeds a threshold, the match is considered “good enough” and the match is formed. We’re constantly experimenting with different match criteria and how to prioritize them.
The matchmaker does not directly try to achieve any particular win rate for players. However, we do try to ensure that each team has a 50% chance of winning in any given match. (This is criteria #1 in the listed above.) We do not examine individual win / loss streaks or try to end them. However, if you are on a winning streak, in general your MMR is probably rising, which will tend to cause you to be matched with higher skilled opponents and teammates. Win rate is not a meaningful measure of player skill.
Win count is also not useful as indicator of skill, and the matchmaker does not use it for that purpose. We do try to group players by their level of experience (criteria #3 in the list above), primarily because we have found that players at the same skill level but different experience level differ in their expectations of how the game is to be played. Our measurement of “experience” for matchmaking purposes is an approximately logarithmic function of the number of games played. The difference in experience between 40 games and 120 games is considered to be about the same as the difference between 120 games and 280.
You can visualize the impact of goals #2 and #3 with a chart where number of games played is the horizontal axis and MMR is the vertical axis. If two players are close together in the diagram, they are considered good candidates to put into a match together. Players who are far apart are considered a poor match. The typical career trajectory of a player new to Dota 2 as he gains experience and moves towards the right is to gradually move upwards as their skill increases. When skilled players create new accounts, they follow a bit different trajectory. Their MMR rises relatively quickly, placing them into the top lefthand corner of the diagram, where they will be matched with other players whose skill is high relative to their experience level.
What About Parties?
When parties are involved, things get a bit more complicated. Parties often contain players with a wide discrepancy in skill and experience. For the purposes of measuring the goodness-of-fit criteria listed as #2 and #3 above, the matchmaker assigns each party aggregate skill and experience numbers. It is these party numbers that are used rather than the individual. In general, when a party with a wide skill range is matched with a solo player, the solo player will have skill and experience near the average of the party. If you notice that one player seems to be significantly less skilled than the other players in the match, it is very likely that they are partied with a high skilled player.
Also, when players are in a party, they typically perform better than players of equivalent skill who don’t know each other. We account for this in two ways. First, we track your skill when queuing alone separately from when queuing in a party. Second, we adjust the effective MMRs based on the number of players in the party and the distribution of skill within the party.
Here’s an example match that was formed today that demonstrates both of these principles in action.
RADIANT DIRE
Party MMR AdjMMR Party MMR AdjMMR
D 2994 3003 C 3046 3062
A 2687 2687 E 2716 2716
F 2788 2788 C 2920 2936
F 2626 2627 B 2672 2672
D 2401 2410 C 2100 2116
TOTAL 13515 TOTAL 13502
Observe that the average adjusted MMR for all of the parties is around 2700. When the players on a team are sorted by adjusted rank, as they are above, the solo players tend to be bracketed above and below by players playing in parties; furthermore, a party with a smaller MMR spread (party F) tends to get bracketed by a party with a larger MMR spread (party D). These patterns are typical. Also notice that party D got a bigger MMR adjustment as a result of the larger MRR spread. Party F, which is formed of players of more equal skill, received a lower bonus. These adjustments were determined using statistical tools (more on this below), but an intuitive explanation is that your performance improves more when partying with a higher skilled player than it does when playing with another player of your same skill.
Data Driven Process
Measuring success in matchmaking is difficult. Players’ appraisals of matchmaking quality are highly correlated with their recent win rate. This includes the members of the Dota 2 team! To avoid emotion and small sample size leading us to “Matchmaking is working well; I’ve been winning”, we try to make design decisions objectively using data. Fortunately, we gather a lot of it. For example, you might wonder how we determined how to adjust effective MMRs to account for the fact that players in a party tend to perform better than players of equivalent skill queuing solo. We used a statistical tool known as logistic regression, which essentially works by trying to create a function that predicts the odds of victory. This function contains several coefficients which determine the MMR bonus given to players in a party. Then we use numerical techniques to solve for the coefficients that produce the function which is most accurately able to predict the match outcome.
Another example of how data drives the matchmaking design process is in deciding when a match is “good enough” and should be accepted, and when we should keep you waiting in hopes of a better match. To help tune this threshold, we start with a measure of match quality. The ultimate goal of matchmaking is fun, and we have several metrics which we use to measure match quality. One such metric measures balance, based on the difference in gold farmed. To be more precise, it’s the time integral of the gold difference, measured since the last point in the game where the difference was zero. This is easily visualized on the gold difference graph. Find the last time when the graph crosses zero, and then measure the area between the horizontal axis and the graph. In general, the smaller this area is, the closer the game was.
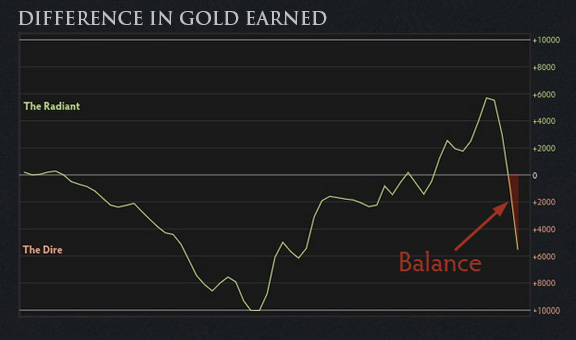
Although at one point in this match the Dire had a 10K gold advantage, the Radiant came back and then pulled ahead, only to have their gold lead reversed again. Despite the fact that at one point in time one team appeared to have a significant lead, our balance calculation judges this match a close game.
Armed with this metric (among others) we have an experimental way to tune the wait time thresholds. We make an adjustment to the threshold, and then observe what this does to the quality of matches, as measured by the distribution of the match balance metric. It’s not critical if the metric misidentifies some edge cases (a game that it measures as close was actually a blowout), since we are typically are only concerned with the aggregate response after making a change.
Conclusion
Hopefully this blog post has given you some insight into how the matchmaker currently works, as well as how we evaluate success and make design decisions. Like most everything else we do, matchmaking is subject to constant reevaluation. Matchmaking will never be perfect, and the technical details in this post refer to the current state of affairs and are likely to change as we find better approaches. We listen to your feedback, and we’re constantly working to improve.
JADI JUTAWAN DENGAN MUDAH
BalasHapusKLIK SAJA laskar4d.net
Bandar Togel Diskon Terbesar di Indonesia Laskar4d.net:
- 2D ( 29% )
- 3D ( 59% )
- 4D ( 65% )
- Game Live Casino, Tangkas, Slot Games, Poker dan Domino
- Discount terbaik serta Rating kemenangan yang Tinggi .
- Minimal Deposit 20.000
Hubungi Kami di :
*BBM : D8EC7434
*WA : +6281 2320 92534
*LINE : laskar4d
*WE CHAT : laskar4d
laskar4d.net